- Published on
How Does StoryDiffusion Achieve Character Consistency in Comics?
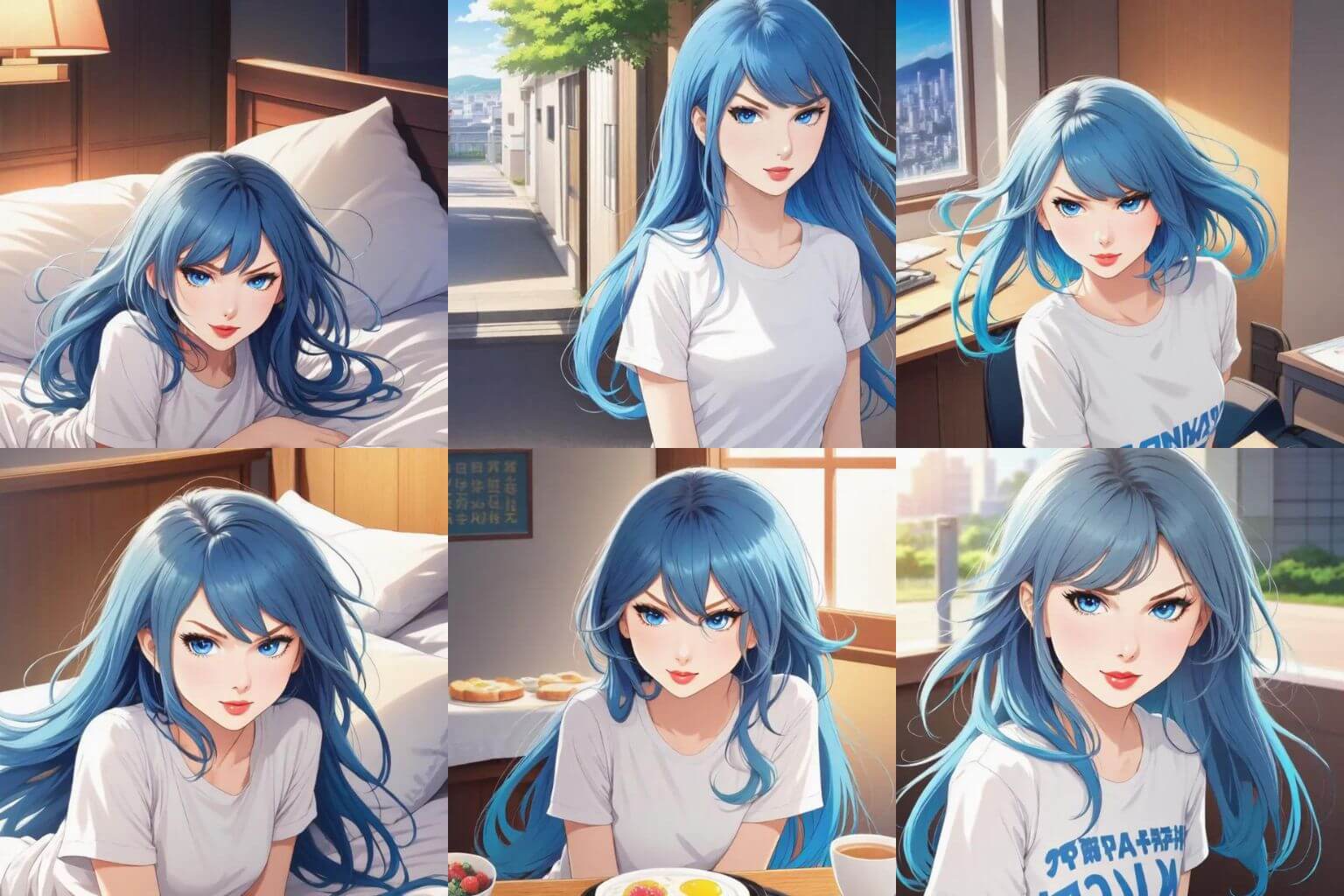
Creating comics with consistent characters is a challenging task, especially when it comes to generating a series of images that tell a coherent story. The latest breakthrough in this field is StoryDiffusion, a novel method that leverages the power of diffusion models to generate not just images, but also videos with consistent characters throughout. Let's dive into how StoryDiffusion achieves this remarkable feat.
Introduction to StoryDiffusion
StoryDiffusion is a state-of-the-art approach that significantly enhances the consistency of characters in generated images and videos. This is particularly important in the context of comic generation, where maintaining the identity and attire of characters across different frames is crucial for the narrative to be engaging and coherent.
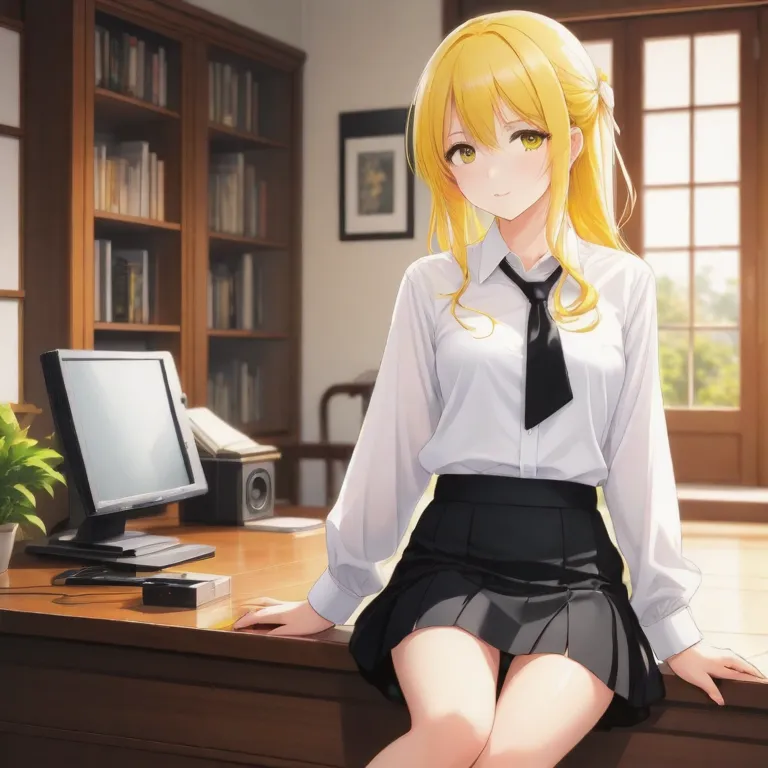
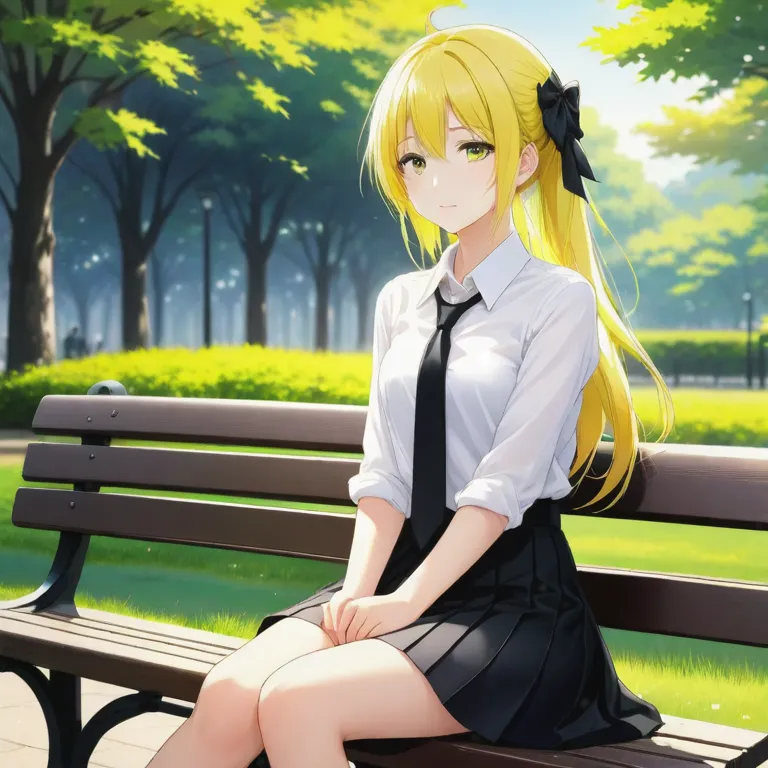
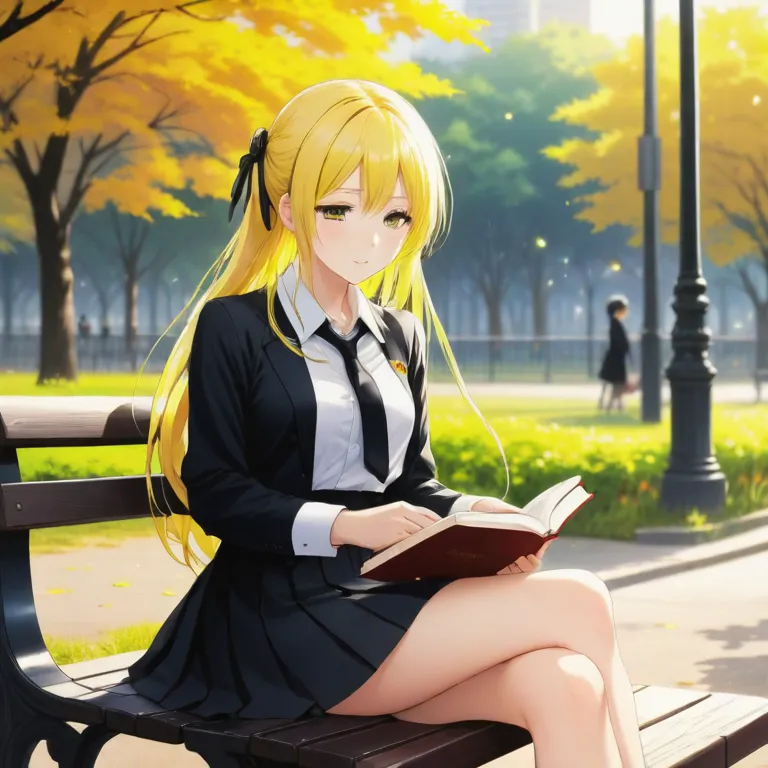
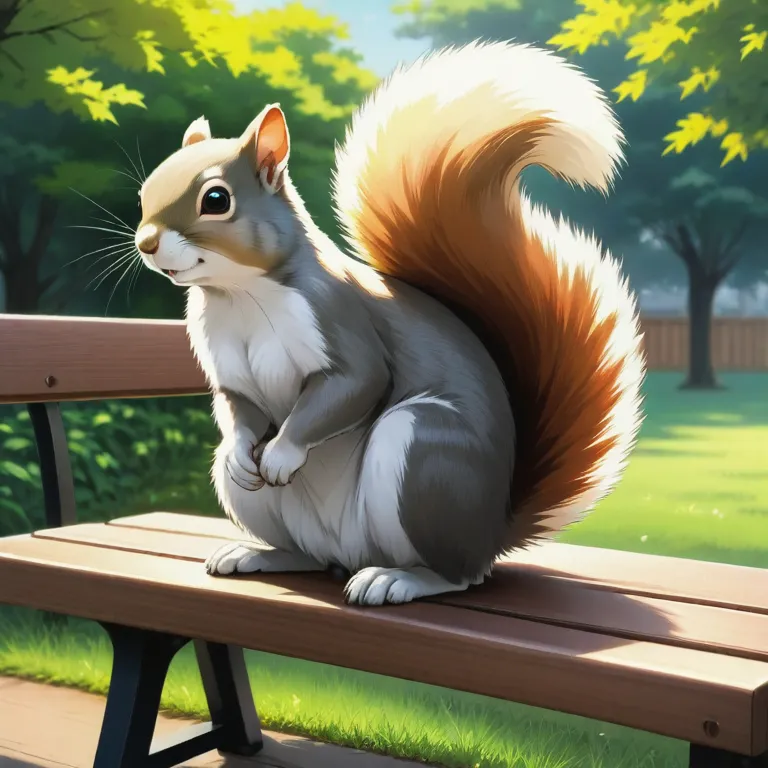
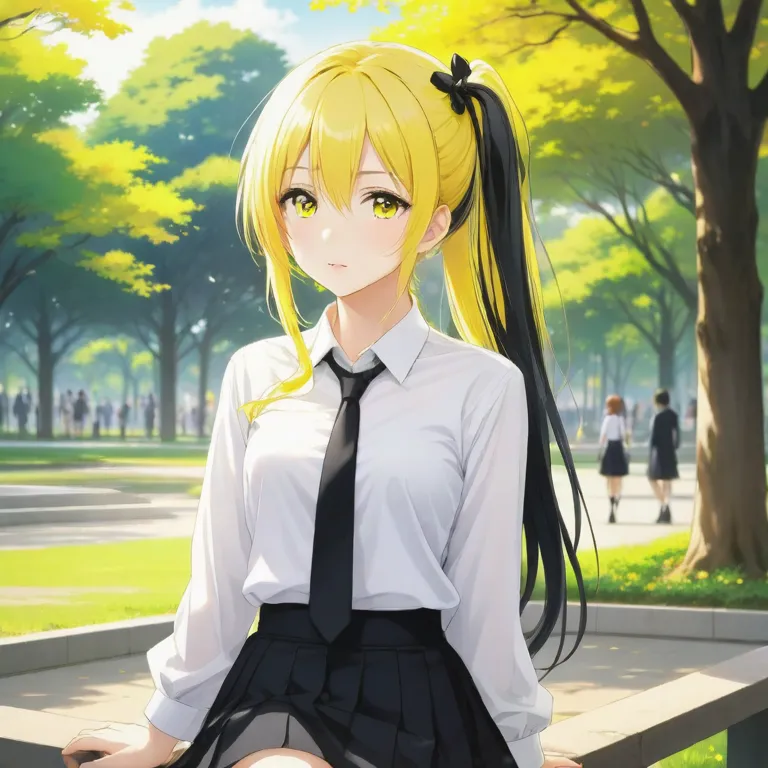
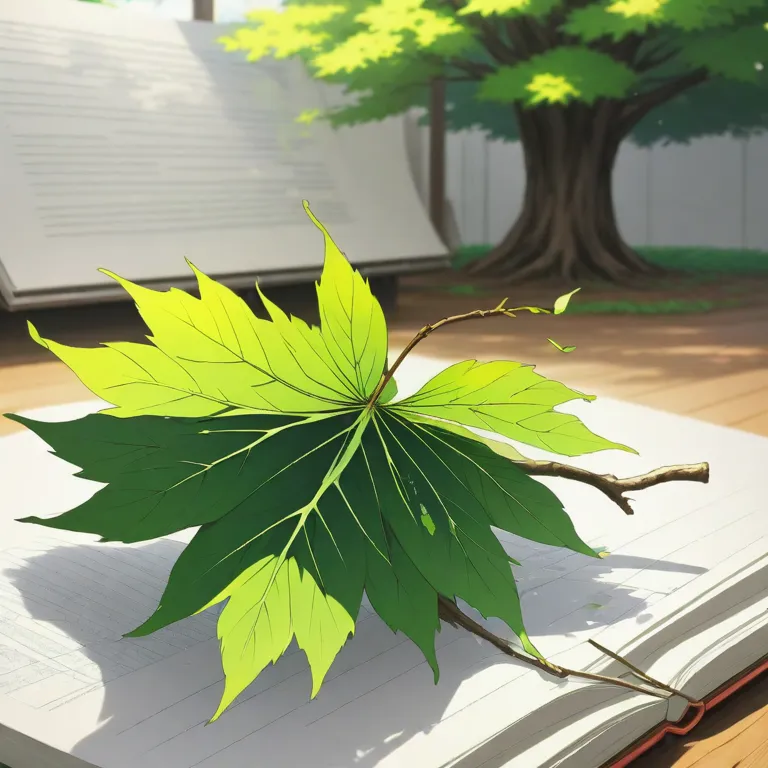
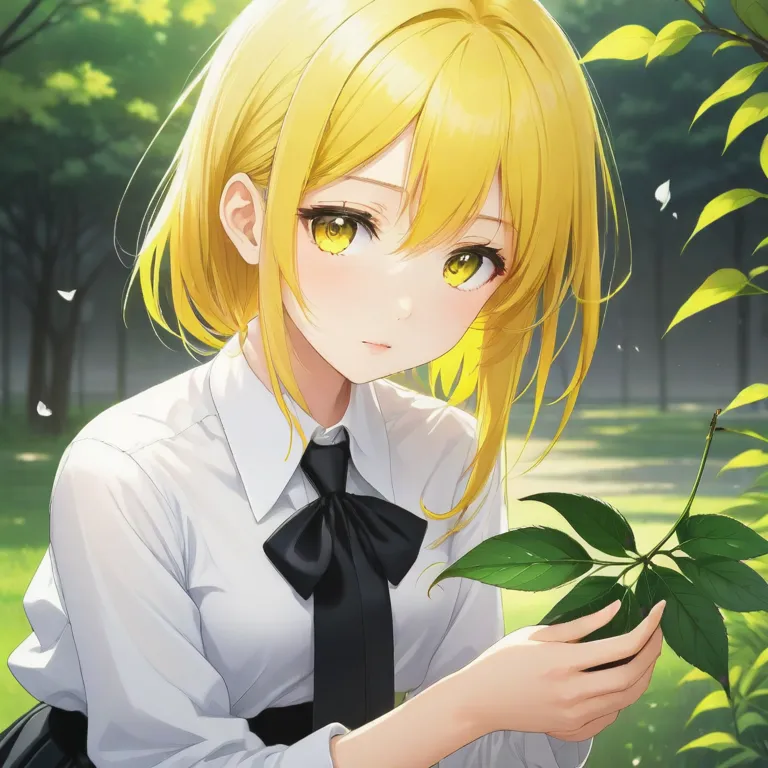
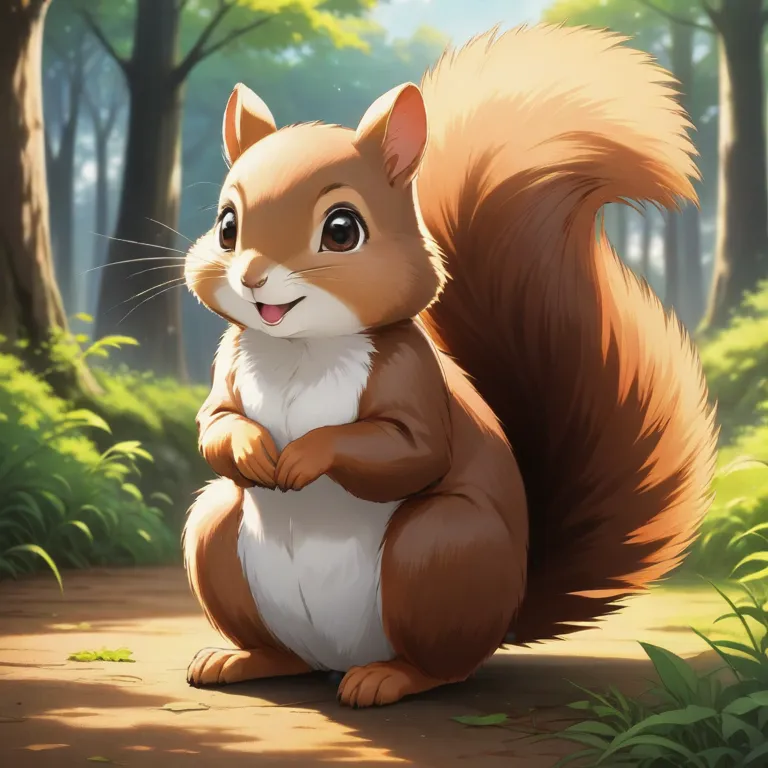
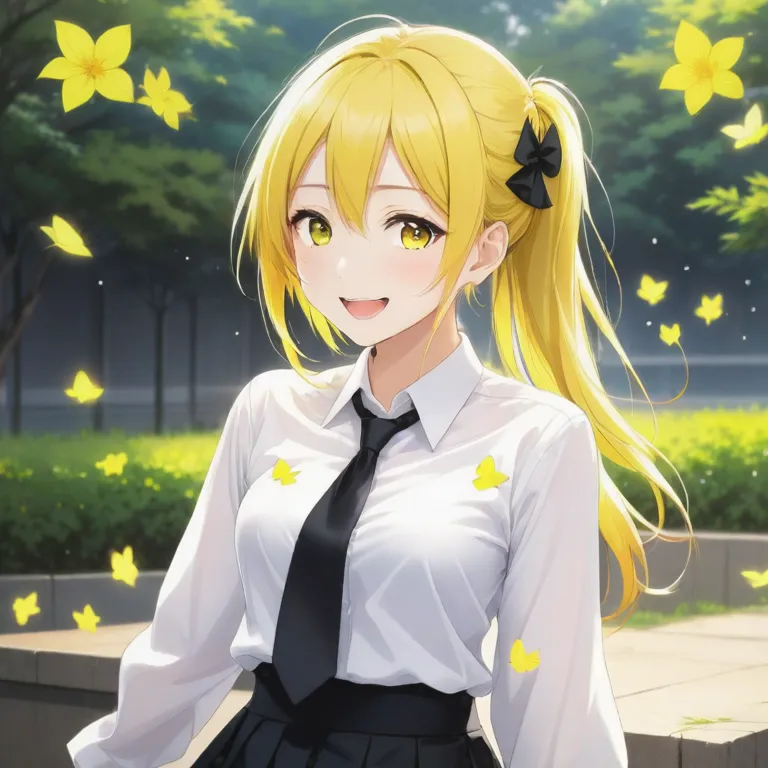
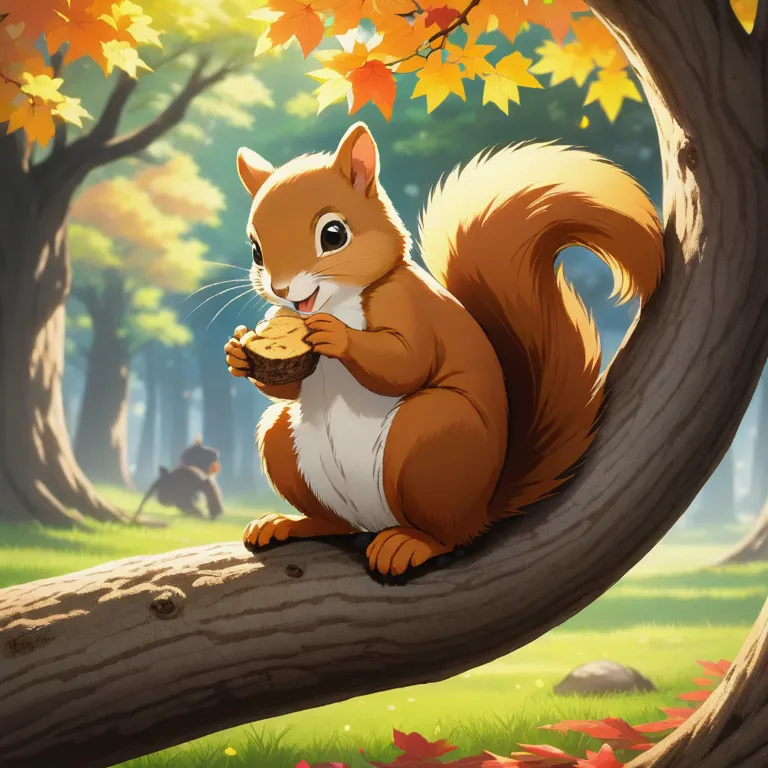
Key Components of StoryDiffusion
Consistent Self-Attention
The core of StoryDiffusion lies in its unique Consistent Self-Attention mechanism. This training-free module is designed to maintain character consistency across a sequence of generated images. It operates by incorporating sampled reference tokens from reference images during the token similarity matrix calculation and token merging. This allows the model to build correlations across images, ensuring that characters remain consistent in terms of identity and attire.
Semantic Motion Predictor
For extending the method to long-range video generation, StoryDiffusion introduces the Semantic Motion Predictor. This module is trained to estimate motion conditions between two provided images in the semantic spaces, converting a sequence of images into videos with smooth transitions and consistent subjects. This predictor works by encoding images into a semantic space that captures spatial information, leading to more accurate and stable motion predictions.
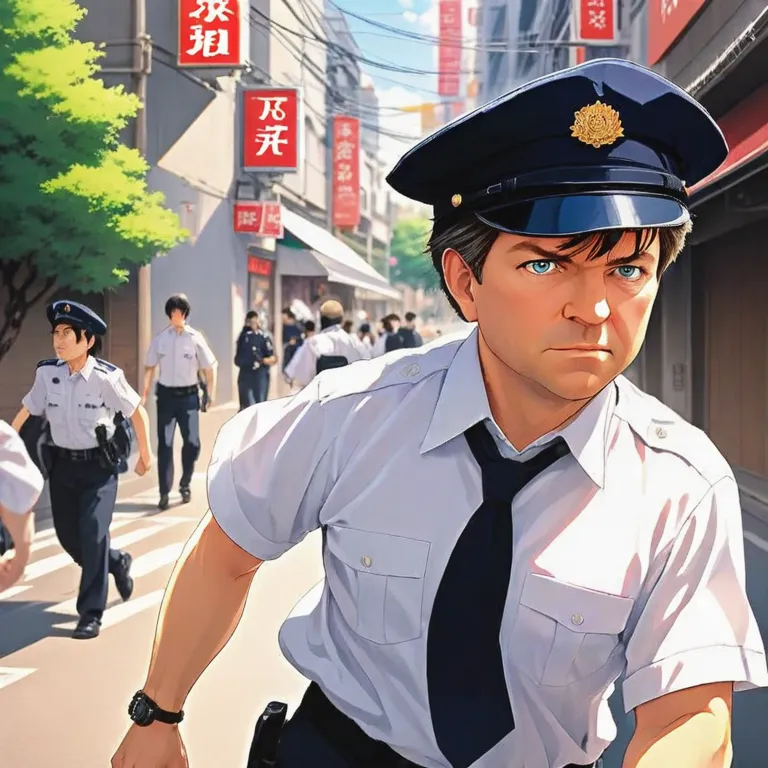
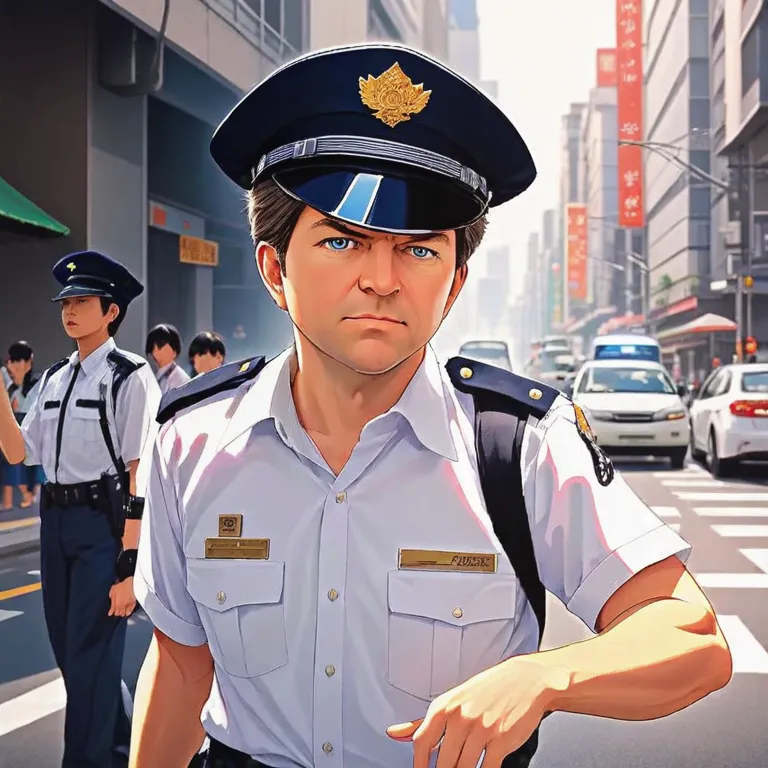
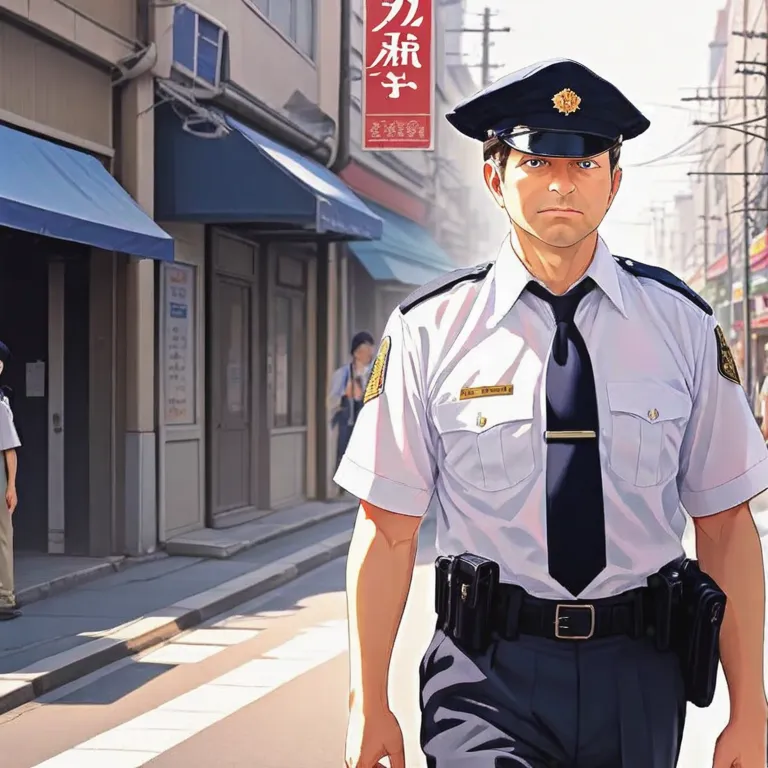
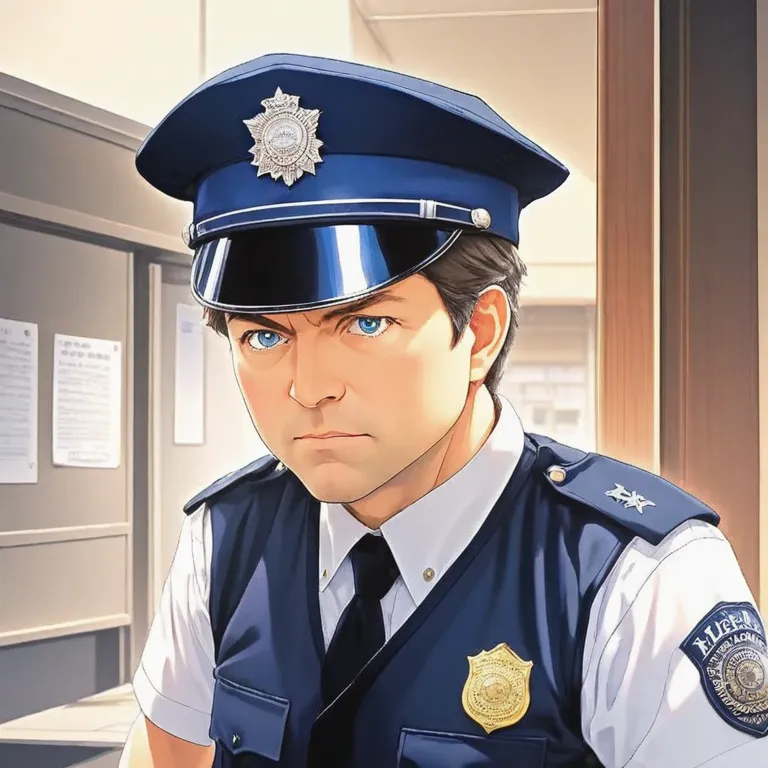
How It Benefits Comic Generation
The combination of Consistent Self-Attention and Semantic Motion Predictor makes StoryDiffusion an ideal tool for comic generation. Here's how:
- Character Consistency: StoryDiffusion ensures that characters maintain their identity and attire across different panels of a comic, which is vital for reader engagement.
- Text Controllability: The model allows for high controllability via text prompts, enabling creators to generate images that closely match their narrative vision.
- Smooth Transitions: In comic strips and animated comics, the smooth transition between frames is achieved through the Semantic Motion Predictor, which generates stable and physically plausible motion.
- Zero-Shot Learning: The Consistent Self-Attention module can be plugged into existing diffusion models without the need for retraining, making it highly adaptable and accessible.
Conclusion
StoryDiffusion offers a new paradigm for generating images, videos, and animations. Are you ready to elevate your storytelling to a new level? Visit StoryDiffusion now and start creating your own animated story!
- Authors
- Name
- Ethan Sunray
- @ethansunray